|
The present study provides the information on the spatial agreement of association between the malaria disease transmissions, mosquito breeding habitats suitability of land use / land cover and weather determinants
|
|
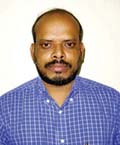 |
M Palaniyandi
|
Digital Mapping Laboratory
|
Vector Control Research Centre,
|
(ICMR) Indira Nagar,
|
Pondicherry, India
|
|
The malaria endemic problem is major concern in the 14 States and Union Territories. The malaria endemic has been severe threaten to the people of highland areas of north eastern states and the eight other states in India, especially in the North-eastern states (Assam, Arunachal Pradesh, Manipur, Meghalaya, Nagaland), Orissa, Chhattisgarh, West Bengal, Rajasthan, Gujarat, Jharkhand, Karnataka, Madhya Pradesh, Uttar Pradesh, Haryana, Maharashtra, Tamil Nadu and Andhra Pradesh. Remote sensing and GIS have been widely used to research works in the developing countries like India where the malaria challenge was the greatest. The study of land use / land cover, climate determinants, the landscape ecology of mosquito breeding habitats and the malaria endemics in different parts of the world including India for the past 40 years (Bhattacharya S, et al., 2006, Dale PE et a., 1998, Hay SI, et al., 1998). Remote sensing and GIS has essentially important role in studying the climate, landscape and the ecological determinants of malaria transmission with reference to space and time (Palaniyandi M, 2012, Liu J and Chen XP, 2006, Ceccato PS, et al., 2005, Sharma VP, et al., 1996, Wood BL et al., 1991 and 1992). The results obtained from the study were provided the information for gaining a better understanding of the spatial distribution of malaria parasitic disease transmission with site specifications at different time points.
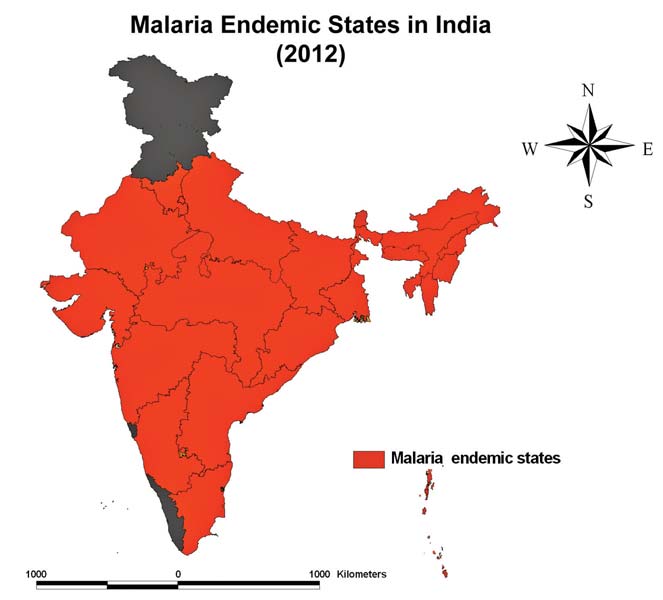
Figure 1: The malaria endemic states / UTs in India as on 2012
The significant studies were made to attribute the spatial relationship between the Land use / land cover changes, geoclimate variables, and the malaria disease transmission (Mushinzimana ES, et al., 2006, Leonardo LR, et al., 2005, Rogers DJ, et al, 2000, Craig, M.H. et al. 1999, Hay SI, et al, 1998, Lindsay SW and Birley MH, 1996, Akhtar R. and Mc Michael AJ, 1996). The specific aquatic habitats with a particular plant communities support malaria vector mosquito breeding habitats (Thomson MC et al., 1996, Wood BL et al., 1991 and 1992). The Normalized Difference Vegetation Index (NDVI) values (-1 and +1) derived from the Multispectral Landsat TM satellite data (Liu J and Chen XP 2006) and, the Indian remote sensing of IRS LISS I and LISS II data were used to analyze the areas of suitability for malaria mosquitoes vector breeding habitats (Palaniyandi M, et al, 2012, Sharma VP et al, 1996). The remote sensing and GIS highbred techniques could provide the relevant surrogate information relating to the spatial variation of the climate variables, the malaria mosquito breeding habitats (Bouma M, et al, 1995, Tyagi BK, et al., 1995, and Wood BL, et al, 1991 and 1992) and the malaria transmission risk. The present study was designed for exploring the use of remote sensing and GIS techniques for mapping malaria transmission risk in India.
Spatial analysis of malaria transmission
The malaria transmission predictive models needs to improve with refining the existing models. GIS was used to construct the thematic maps, spatial analysis and the spatial modeling of malaria transmission risk as perennial, seasonal, bi-seasonal, sporadic epidemics etc., perhaps, GIS allow layers of information of malaria vectors and climatic variables (Temperature, relative humidity, saturation deficiency and rainfall). The indicator of malaria stability is the reproduction rate (Ro) of the disease: when Ro is less than this would be a Boolean situation, where climate is suitable (1) or unsuitable (0). Defining Boolean thresholds above which the temperature–rainfall combination is considered suitable and where malaria is expected to occur, or below which malaria is expected not to occur, would be ignoring natural gradients and inherent uncertainty. The digitally superimposed thematic maps of climate variables with the malaria epidemic cases for performing the spatial analysis to construct a spatial modeling for predicting malaria transmission risk in the endemic and non-endemic areas (Small et al., 2003, Craig, M.H. et al. 1999, Akhtar R. and Mc Michael AJ, 1996, Hay SI, et al, 1998, Lindsay SW et al., 1996 and 1998, Martens P, et al., 1999). The results were provided that the geographical distribution of malaria transmission and spatial diffusion are greatly in?? uenced by geo-climatic variables.
Land use / land cover changes and malaria epidemics
Land use / land cover changes (dry land agriculture to irrigation wet cultivation) and the growth stage of vegetation types may perhaps play an important role in determining vector abundance. The irrigation rice cultivation ?? elds have been provided breeding sites for Anopheles gambiae early in the growth cycle of the plants, these changes as the rice plants mature and form a dense canopy cover over the water (Wood BL, et al., 1991 and 1992). The combination of regional climate changes (temperature, rainfall, and humidity) and land use / land cover changes have been fueled to promoting the vector borne disease epidemics in newer areas (Bouma M, et al, 1995, Tyagi BK, et al., 1995). The recent years has been witnessed by growing incidence of vector borne diseases in different parts of our country, and more frequently in the districts where the irrigation water resource projects were brought out, and these factors have fueled for malaria vector mosquito abundance around of the buffer zone of 2.5 Km of the irrigation wet cultivation agriculture areas (Palaniyandi M, 2004, Tyagi BK, et al, 1995).
Weather determinants and malaria transmission
The meteorological variables (Temperature, humidity, saturation de?? ciency, and rainfall) were used for predicting the area in severity of malaria transmission risk (Bhattacharya S, et al, 2006, Singh N and Sharma V P, 2002, Craig, M.H. et al. 1999, Lindsay SW, et al, 1996, Martens P, et al., 1999, Akhtar R. and Mc Michael AJ, 1996). The conventional method of malaria transmission risk assessment based on the epidemiological data may cause error, however, the remote sensing, and GIS techniques, a malaria risk map could be prepared based on the geo-climatic variables provides the guidelines for designing strategic plan for control of malaria epidemics. Based on the previous studies, it was well established facts that climate determinants are being most important in limiting the malaria transmission.
Temperature and rainfall effects on malaria transmission
Temperature has been playing the most significant role in the determinants of the vector survival, parasite development, parasite incubation, and the malaria disease transmission. The optimal temperature range for Anopheles vector species of malaria development lies within 20°C to 30°C. However, transmission of P. vivax requires a minimum average temperature of 15°C and transmission by P. falciparum, requires a minimum temperature of 19°C. The effects of temperature on the transmission cycle of the malaria parasite Plasmodium falciparum and Plasmodium vivax has manifold, but it has specific and significant effects on duration of parasite development (n) and mosquito survival (p) are the most important (Bhattacharya S, et al, 2006). The spatial agreements and the association between the malaria vector abundance and rainfall is most complex and the predictable relationship does not exist. There is no clear relationship observed between the positive malaria cases and the annual precipitation alone (Bhattacharya S, et al, 2006). The amount of rainfall, the number of rainy days and the degree of wetness has no significant effects on malaria prevalence.
Relative humidity and saturation deficiency
The rainfall and humidity are important determinants of vector breeding and survival, parasite developments and the spatial diffusion of malaria transmission. There were many pioneers carried out studies on the influence of climatic conditions with respect to malaria transmission in the arid and semi-arid areas (Bouma M and Van Der Key HJ, 1995 and Tyagi BK, et al, 1995). Rainfall and humidity on vector populations and, the influence of sturdiness of precipitation on malaria transmission current scenarios was most associated with types of landscape environments (Singh N and Sharma VP, 2002). The climatic condition of relative humidity with range between 55 per cent and above 80 per cent could provide the suitable environment for malaria transmission (Bhattacharya S, et al, 2006).
The cumulative effects of climate variables on malaria transmission
The spatial relationship between the climate variables and the Anopheles genus malaria vector shows that the model fitted with statistically significant. The most Anopheles vector species of malaria, the optimal temperature range for their development take place within 20°C to 30°C. However, transmission of P. vivax requires a minimum average temperature of 15°C and transmission by P. falciparum, requires a minimum temperature of 19°C. It has been observed in India, that the P. vivax vector requires 15 to 25 days to complete its cycle if the temperature remains within 15°C to 20°C, the relative humidity for both species remains within 55 to 80% and its life cycle maybe get completed even within 6 to 10 days, if the temperature range remains within 25°C to 30°C. The relative abundance of the malaria vectors are directly controlled by the climate variables (Figure 2). The multivariate geostatistical model predicted accurately the spatial association between the malaria transmission and climate variables, and perhaps, supplementary tool for assessing the relative abundance of malaria vectors breeding habitats suitability in the country with accuracy of 85% and improved to 90% (Table.1). The geostatistical analysis of linear multivariate analysis provides the strong relationship between the geo-climatic variables and malaria transmission. The longevity and survival of infected mosquitoes and the prevalence of the disease have been spatially associated and are definitely controlled by the geoclimatic variables. The malaria epidemics in India has spatially significant with the range of population density, it means the village settlement clusters within the range of population from 100 to 1100 was mostly affected by malaria transmission across the country (figure 3a and figure 3b). The multivariate analysis has statistically significant when the variables were analyzed simultaneously. The result was arrived to demarcate the boundary of the malaria risk zones in the country, based on the Malaria Transmission Risk Index (Figure 4). The predicted malaria transmission risk zones and the community of people at of malaria transmission could be datum of baseline for decision making and disease control in the country.
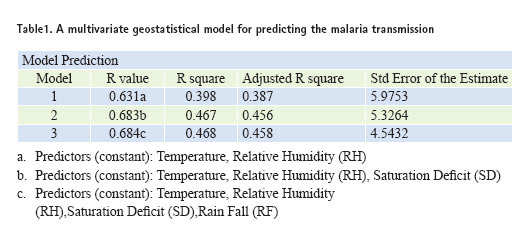
Remote sensing and GIS for mapping malaria transmission
Remote sensing and GIS has the important role in mapping the ecological aspects of Anopheles genus malaria vectors breading habitats. Remote sensing of IRS LISS I and LISS II data products were integrated into GIS for spatial analysis for classi?? cation and mapping of land use land cover categories and mapping the breading habitats of Anopheles genus vector mosquitoes (Palaniyandi M, 2012, Sharma VP, et al, 1996). The spatial relationship between the normalized differential vegetation index (NDVI) and the Anopheles genus malaria vector shows that the model fitted with statistically significant (Liu J and XP Chen, 2006). Subsequently, it helps to assess the people at risk of malaria epidemic in the different environments of geographical areas for delineating the malaria epidemics well in advance. The large data sets of climatic variables were integrated with remote sensing data for mapping the seasonality and the occurrence of malaria transmission intensity. The Normalized Difference Vegetation Index (NDVI) derived from the satellite data ranges between the value of (-) 1 and (+) 1. The spatial agreement between the observed and predicted values of logistic regression model is r=0.76 sensitivity and specificity of 0.78 of larval index within a buffer around the trap location of rice fields (Wood BL, et al, 1991 and 1992). The higher NDVI values (correlated with high rice tiller densities) in the early growing season were found to have higher larval mosquito densities (Leonardo LR, et al., 2005). The remote sensing information was integrated with human settlements and livestock data in the linear multiple discriminant analysis enabled added advantage of mapping the host availability of anopheles genus breeding habitats suitability.
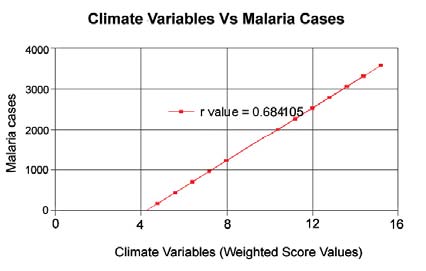
Figure 2: The spatial relationship betweenthe climate variables and the occurrences ofmalaria epidemic cases across the country
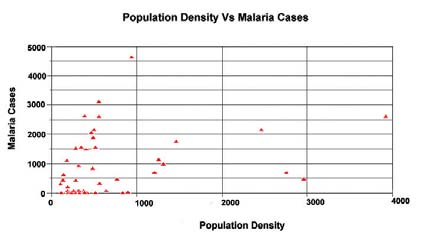
Figure 3a: The spatial relationship between thepopulation density in the settlement clusters with rangeof 1000 to 4000 and the malaria cases in India
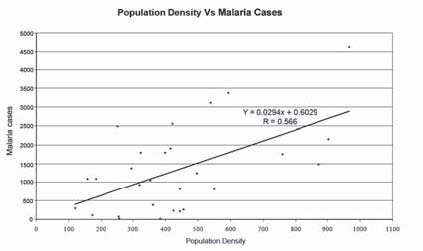
Figure 3b: The spatial relationship between the PopulationDensity or village settlement clusters with the populationrange of 100 to 1100 and the malaria cases in the country
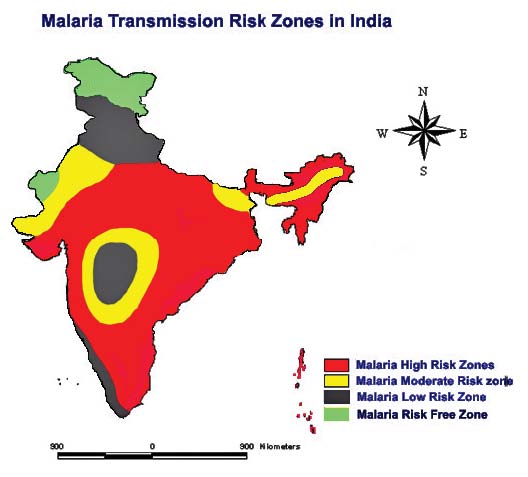
Figure 4: The malaria transmission risk zones inthe country based on the climate variables
Conclusion
Remote sensing and GIS were used for mapping the malaria vector breeding habitats facilitate to estimate the people at risk of malaria transmission. The results show that spatial agreement was existed between the environmental variables and the malaria endemics. The remote sensing and GIS has been provided the guide lines for mapping transmission risk zones based on the information derived from the geostatistical analysis of environmental variables in the country. Thus, the techniques could be used as the datum of baseline for prioritizing the areas for taking appropriate prevents measure to the malaria transmission control in the country.
References
Akhtar R. and Mc Michael AJ, 1996. Rainfall and malaria outbreaks in Western Rajasthan. Lancet, 348: 1457–1458
Bhattacharya S, et al, 2006. Climate change and malaria in India, Current Science, 90(3): 369 – 375.
Beck LR, et al., 1997. Assessment of a remote sensing-based model for predicting malaria transmission risk in villages of Chiapas, Mexico. Am. J Trop. Med. Hyg. 56: 99–106
Bouma M, et al, 1995. Epidemic malaria in India’s Thar Desert. Lancet 346: 1232–33
Ceccato PS, et al., 2005. Application of geographical information systems and remote sensing technologies for assessing and monitoring malaria risk. Parassitologia 47(1): 81-96
Craig, M.H. et al. 1999. A climatebased distribution model of malaria transmission in sub-Saharan Africa. Parasitol. Today 15, 105–111
Dale PE, et al., 1998. An overview of remote sensing and GIS for surveillance of mosquito vector habitats and risk assessment. J Vector Ecol, 23: 54-61
Hay SI, et al, 1998. From Predicting Mosquito Habitat to Malaria Seasons Using Remotely Sensed Data: Practice, Problems and Perspectives Parasit. Today, 14(8): 306 – 313
Hay SI, et al., 1998. Prediction of malaria seasons in Kenya using multi-temporal meteorological satellite sensor data. Trans. R. Soc. Trop. Med. Hyg. 92, 12–20
Leonardo LR, et al., 2005. A study of the environmental determinants of malaria and schistosomiasis in the Philippines using remote sensing and geographic information systems. Parassitologia 47 (1):105-14
Lindsay SW and Birley MH, 1996. Climate change and malaria transmission. Ann. Trop. Med. Parasit. 90, 573–588 resurgence – reply. Nature 420: 628
Liu J and XP Chen, 2006. Relationship of remote sensing normalized differential vegetation index to Anopheles density and malaria incidence rate. Biomed Environ Sci 19 (2):130-2
Mushinzimana ES, et al., 2006. Landscape determinants and remote sensing of anopheline mosquito larval habitats in the western Kenya highlands. Malaria J 5(1):13
Palaniyandi M and T Mariappan, 2012. “Remote Sensing and GIS for malaria control in the urban environment- a case study, Vizagappattinam City, India”, Geospatial World, 27th Feb, 2012 Vol.8 Issue 9, PP1-9
Roberts JF, et al., 1994. Remote sensing as a landscape epidemiologic tool to identify villages at high risk for malaria transmission. Am J Trop Med Hyg 51(3): 271-80
Sharma VP, et al., 1996. Study on the feasibility of delineating mosquitogenic conditions in and around Delhi using IRS satellite data. Indian J. Malariology 33: 107–125
Singh N and Sharma VP, 2002. Patterns of rainfall and malaria in Madhya Pradesh, central India. Ann. Trop. Med. Parasitol., 96(4): 349–359.
Small J. et al., 2003. Climatic suitability for malaria transmission in Africa, Proc. Nat. Acad. Sci. USA, 100: 15341–15345
Thomson MC. et al., 1996. The ecology of malaria – as seen from earth-observation satellites. Ann. Trop. Med. Parasit. 90: 243–264
Tyagi BK, et al.,. 1995. Epidemic malaria in Thar Desert. Lancet 346: 634–35.
Wood BL, et al., 1992. Estimating high mosquito-producing rice fields using spectral and spatial data. Int. J. Remote Sensing, 13, 2813–2826
Wood BL. et al., 1991. Spectral and spatial characterization of rice field mosquito habitat. Int. J. Remote Sensing 12: 621–626 |
Leave your response!